All Science News
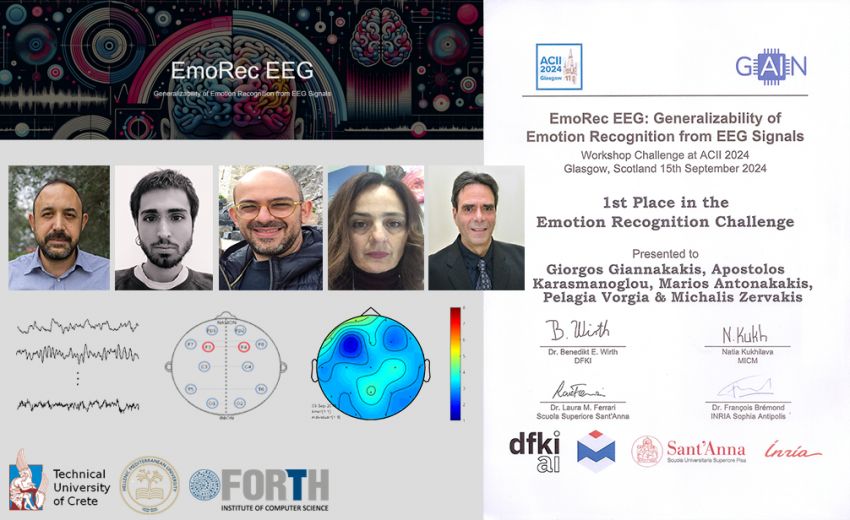
1st Place Award in the international emotion recognition challenge from EEG signals
A research team from the Technical University of Crete, the Hellenic Mediterranean University (HMU), and the Institute of Computer Science (ICS) of the Foundation for Research and Technology - Hellas (FORTH) won the 1st place award in an international emotion recognition challenge using electroencephalography (EEG).
The competition took place within the framework of the recognized 12th International Conference on Affective Computing & Intelligent Interaction (ACII), held in Glasgow, Scotland, from 15-19/09/2024 (https://acii-conf.net/2024). An open international competition (https://emotionlab.github.io/EmoRec-EEG/challenge) was organized to recognize the underlying emotion through publicly available EEG data. Five research teams and 13 researchers participated in the competition, aiming to find the most efficient method for emotion estimation from the data.
The research team included Giorgos Giannakakis (Associate Professor at the Department of Electronic Engineering, HMU and Research Associate at the Institute of Computer Science, FORTH (https://users.ics.forth.gr/~ggian), Apostolis Karasmanoglou (MSc student at the Technical University of Crete), Marios Antonakakis (Research Associate at the School of Electrical Engineering, Technical University of Crete, Pelagia Vorgia (Pediatric Neurologist, Researcher at the Institute of Life Sciences, HMU) and Michalis Zervakis, Professor at the School of Electrical Engineering and Rector of the Technical University of Crete (https://www.display.tuc.gr).
The methodology followed included evaluating the most relevant EEG features related to the emotion under investigation (such as common spatial patterns, Hjorth parameters, brain asymmetry, beta/alpha power ratio, and normalized brain rhythms) and then applying automatic classification using artificial neural network architectures.